Amanda Chaboryk and Nicholas Cook highlight the importance of reviewing your information architecture before using your data for generative AI
The advent of artificial intelligence (AI), and generative AI (GenAI) in particular, has resulted in a dawning era of transformative potential in the legal domain. It has been met with a range of reactions from fervent anticipation to cautious circumspection. As with any disruptive technology, the integration of AI into legal practice is a delicate balance of innovation and introspection, resulting in adoption challenges almost as diverse as the challenges the technology promises to address. To enable the adoption of AI within a given legal team, there are a range of factors that need to be present. These include the availability of suitable legal data sets, subject matter experts in both law and technology, and robust AI governance structures. These elements form an organisation’s ‘information architecture’ (IA) and are a necessary precursor to successful AI deployments.
How did we get here?
It’s helpful to pause and ask ourselves ‘How did we get here?’, to determine what has led to the greater availability of AI technologies, specifically the plethora of emerging GenAI tools.
GenAI has been identified as one of the greatest disruptors to the modern legal industry (as well as being similarly disruptive to wider knowledge and professional service industries, and beyond). Advances in AI, particularly the ‘transformer’ architecture, have been revolutionary, enabling models to process sequential data (such as natural language) more effectively, and for us to build larger and larger models that benefit from this technique. As detailed in the Google research paper “Attention is all you need” (2017) (see also a summary here), transformers use self-attention mechanisms to weigh the importance of different parts of the input data / text, allowing for a more nuanced understanding, interpretation and generation of complex context-specific output text. Attention was not a new concept in 2017. However, the way it was used in the pivotal research paper turned traditional thinking on its head, dispensing with predecessor recurrent neural network and convolutional neural network techniques to instead use only ‘self-attention’ (which could benefit from vectorisation techniques to work far faster and more effectively). OpenAI built on the paper to release GPT-1 (2018), GPT-2 (2019) and GPT-3 (2020), none of which captured mainstream attention (pardon the pun). It was not until the release of ChatGPT in November 2022, a chatbot that initially used GPT-3, that the global zeitgeist took notice of the transformer architecture, large language models (LLMs) and GenAI more widely. Today, all current generation LLMs use the transformer architecture under the hood.
The 2017 Google paper was focused on the impact of the transformer and how it could improve machine translation. However, these developments have resonated more widely across other markets and industries, including the legal profession. Transformers can analyse large amounts of text efficiently and their capabilities continue to increase. For instance, Anthropic’s Claude 3 can handle a context of 1 million tokens or approximately 700,000 words. This efficiency enhances the accuracy of document review and legal research by understanding context and nuances, at least for those users properly trained in prompt engineering techniques and familiar with the constraints of these systems. A token, in the context of LLMs, is a unit of data (like a word, or part of a word) that the LLM uses to understand and generate text. Such accuracy is, of course, crucial in legal work where the significance of words and patterns, can greatly impact case outcomes and legal interpretations: think of ‘may’ vs ‘shall’ vs ‘must’, or ‘undertakes’ vs ‘reasonable endeavours’ vs ‘best endeavours’. Transformers, using attention mechanisms to weigh the importance of different parts of the input data (prompt), allow for more nuanced understanding and generation of complex patterns, particularly from the increasing number of models that have received further legal specific fine tuning.
These technologies can also be used in the context of outcome prediction in disputes, provided there is availability and sufficient granularity of rich legal data sets (which will be explored in more detail below). The developments discussed above have led to major improvements in natural language processing (NLP) tasks, resulting in GenAI now being able to produce highly coherent and contextually relevant text (even images and other media), with broad applications across all industries – but particularly for legal services. GenAI is likely to effect an imminent and ongoing democratisation of machine learning (ML). Historically, training ML algorithms/models has required tens of thousands of pre-labelled data pairs. Market-based access to data has lowered entry barriers and led to an abundance of new AI applications. Using an LLM, what once took a team of ML engineers and developers weeks, and datasets of tens of thousands of labelled examples, can now be achieved in an afternoon by a single human with a computer and internet access, relevant model API keys (or an open-source model), and a bit of flair with multi-shot prompt engineering. This democratisation of ML has empowered individuals and smaller companies, as well as law firms, in-house legal and legaltech teams, who can now establish proofs of concept and progress to fuller deployments across their organisations.
Data Availability and Cleaning
All modern AI systems are built on a foundation of data. For AI to be effectively adopted within a law firm or legal team, the AI must have access to comprehensive legal data sets. These data sets should include data such as historical matter files, document precedents, legislation, regulations, guidance papers, case law, and other relevant legal documents. The quality of these data sets is paramount. They must be free of errors, accurate, up-to-date, and representative of the diverse range of cases the legal team may encounter. Moreover, the data must be structured in a way that is accessible and understandable by AI systems, which often requires significant data cleaning, preprocessing and standardisation efforts. While many law firms already employ knowledge management teams, fewer have data stewards or dedicated teams tasked with maintaining mission critical data sets and data warehouses (although this is changing, extremely rapidly, as law firms race to embrace this tech).
Legal datasets in all jurisdictions must adhere to applicable data protection laws, such as the European General Data Protection Regulation (EU GDPR) and, in the UK, the General Data Protection Regulation (UK GDPR) and the Data Protection Act 2018 (subject to any reform that may occur should the Data Protection and Digital Information Bill ultimately pass into law). In any event, these laws require a lawful basis for processing personal data, maintaining data accuracy, implementing data minimisation, ensuring confidentiality, and granting data access rights. We are also starting to see the emergence of a new role of AI governance professionals who are working to help organisations understand and comply with the EU AI Act and other legislation emerging globally. There are clear analogies for what is happening now with AI, and what has happened since 2016 in respect of the EU GDPR in respect of data privacy.
To harness AI effectively at scale within a legal team, a robust infrastructure is paramount. This infrastructure must encompass well-organised, accessible, and annotated legal data to support the development of expansive models and AI tools. This foundation necessitates a holistic examination of business processes, ensuring AI integration enhances the entire workflow rather than isolated segments. This can be concisely described as ‘information architecture’ (IA) (also known as ‘data infrastructure’). IA in the context of artificial intelligence refers to the deliberate organisation and structuring of data and information systems to optimise their usability and effectiveness. This enables AI algorithms to effectively parse, understand, and learn from data, which is crucial for tasks such as pattern recognition, decision-making, and predictive analytics. Effective IA is essential for AI to access relevant data efficiently and to scale its capabilities, ultimately enhancing the performance of AI applications and systems.
The process of data cleaning and curation, while key, is often overlooked, and serves as a barrier to entry for aspiring legal technologists. Gartner recently reported that at least 30% of GenAI projects currently ongoing will not proceed beyond proof of concept by the end of 2025 for a variety of reasons such as poor data quality, inadequate risk controls, ambiguous business value, and escalating costs. Within the legal sector, the issue of data management takes on an additional layer of complexity. Data in legal teams often remains siloed in various departments across the business. For example, financial data, which can provide rich insights on matter profitability (such as write-offs, growth areas of business, phase and task code metrics, and other insights) is often trapped within the finance department. Matter data, which starts its ‘information’ lifecycle on matter opening forms for compliance and audit purposes, is often not even connected to financial management systems directly. These data sets can be reconciled, for example, by cross referencing the project’s matter number within the matter opening system to the finance system. However, this requires a reconciliation exercise which can often be manual and inefficient. The current state of legal data management is reminiscent of the embryonic stages of data science prior to the widespread adoption of databases. To take full advantage of AI, organisations may ultimately have little choice but to upgrade their IA and integrate their data processes and behaviours.
In another context, legal data management poses distinct challenges for public sector organisations, who must manage high volumes of cases, integrate diverse data sources and strictly comply with public & administrative law requirements (in addition to legal and privacy requirements of general application), all whilst in the glare of the public eye, and often with limited budget and resource. Consider the judiciary as an example of this, where there is a growing backlog of cases, illustrating the severe strain on our system of justice: 347,820 active cases in magistrates’ courts and 62,766 active cases in Crown Courts as of September 2022; and 1.6 million civil claims and 266,000 family court claims commenced in 2021. Likewise, the rising median magistrates’ court waiting time of 196 days highlights the imperative to take action, for example by adopting digital solutions and AI tools. We must try to alleviate pressure, enhance efficiency and reduce delays. As the legal maxim goes, “Justice delayed is justice denied”; individuals who do not receive timely legal recourse may be subject to adverse impact to their employment, economic and housing circumstances, and personal relationships. In criminal cases, defendants may be held in custody for extended periods without a verdict, raising concerns about the presumption of innocence and the right to a fair trial. Conversely, the size of the court backlog in England & Wales may actually offer an opportunity to leverage data to pinpoint inefficiencies and streamline processes. To facilitate this, we need accurate and well-organised data, as this is essential for ensuring fair and timely access to justice. Such data enables courts and legal professionals to better manage cases efficiently, prioritise urgent matters, and make informed decisions.
When data is accurate and easily accessible, it reduces the likelihood of errors, delays, and missed deadlines, which can otherwise prolong legal proceedings and undermine the fairness of outcomes. It also enables predictive analytics in court case management, using historical data and machine learning algorithms to forecast the likelihood of outcomes (such as case duration, or the potential for settlement). By analysing factors like case type, complexity, and past decisions, AI can help decision makers to triage and prioritise cases that are more likely to require immediate attention or those at risk of significant delays. This approach could enable courts to allocate resources more efficiently, ensuring that urgent and high-impact cases are processed faster, ultimately helping to reduce the overall backlog and improve access to justice.
By focusing on data quality, interdisciplinary collaboration, and AI integration, the public sector can harness AI tools to bridge gaps in access to justice and ensure timely, equitable legal outcomes. However, this naturally requires a combination of legal and technical subject matter experts (SMEs) working collectively to translate technical requirements into operational outcomes, and vice versa.
Multi-disciplinary Teams
The intersection of law and AI technologies (ML, NLP, GenAI, etc.) is highly specialised, necessitating the involvement of SMEs who possess expertise in both domains. Legal SMEs understand the nuances and complexities of the law, whilst ML SMEs are adept at building, training, and refining AI models and tools. The collaboration between these two panels of experts is crucial for developing AI tools that are both legally sound and technologically effective. Moreover, experts in AI regulation and data privacy law are required to advise on the permissibility of, and constraints on, using these technologies. They need a deep understanding of the likes of web scraping and intellectual property law as well as principles of responsible AI, such as AI that is helpful, honest and harmless. These professionals together bridge legal teams and technologists, ensuring that the AI’s outputs are interpreted correctly and applied appropriately within a legal domain. This is what is meant by ‘AI governance’, a new emerging sub-field in its own right, at the intersection of law and compliance on the one hand, and AI and tech on the other. Implementing AI with a legal team demands robust governance frameworks to ensure ethical, transparent and accountable use of AI. This includes establishing clear policies for data privacy, security and usage, as well as setting standards for AI performance and accuracy. AI governance also involves creating mechanisms for monitoring the AI systemic decision-making processes, auditing processes, and providing recourse for any errors or bias that may arise. It is essential that the users (legal teams, and ultimately any clients or internal stakeholders) have confidence in AI outputs, and that there are procedures in place to address any failures or issues that may occur.
Conclusion
When deploying an AI strategy, the project team needs to recognise AI as a critical infrastructure layer, which must be integrated into the overall strategy, with a focus on its effects on business models. Analytics enabled by AI serve as a great data mining machine for legal teams, unearthing all kinds of correlations and previously hidden patterns. The adoption of AI within a legal team requires a cultural shift and openness to change. Legal professionals need to retool and be willing to adapt to and adopt new technologies and embrace the potential of AI to transform legal practice. This often requires change management strategies (and professionals) to address potential scepticism or resistance, highlighting the benefits and addressing the challenges. Education and awareness programs can help demystify AI for legal professionals and demonstrate its practical applications in their daily work. All of us are already living in a brave new world of legal tech and GenAI. However, not all of us have realised this yet, and are letting the bandwagon pass them by. Without wishing to over-pander to hype or the FOMO, one does have to wonder whether that bandwagon will be full by the time they seek to get on board.
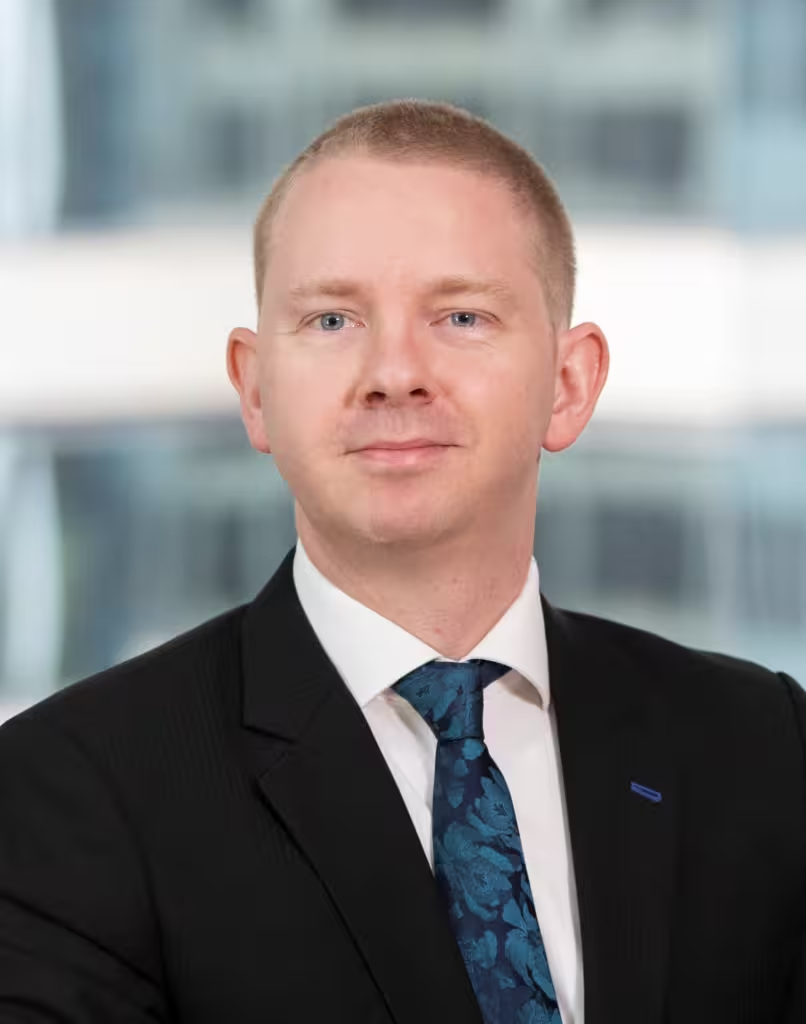
Nick Cook is a solicitor and certified Artificial Intelligence Governance Professional (AIGP, IAPP). He sits on PwC China’s AI Strategy Taskforce, and is the PwC project driver and deployment lead for Harvey AI in Greater China.
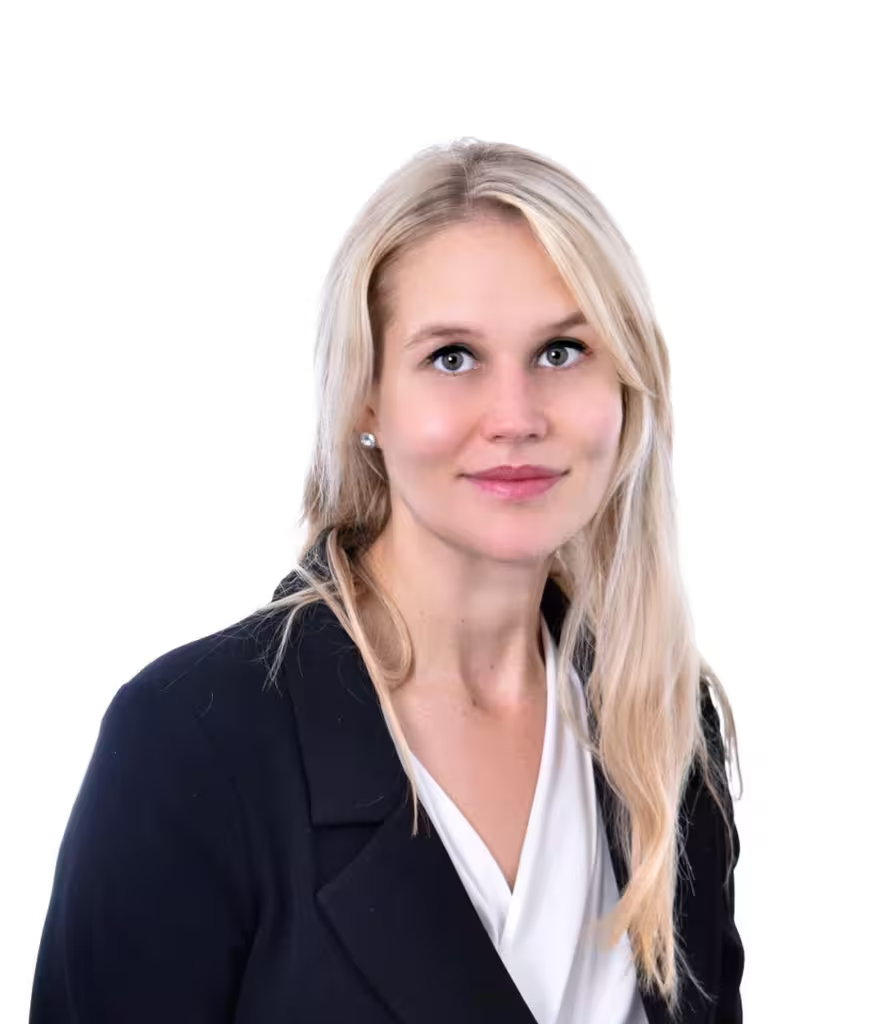
Amanda Chaboryk is the Head of Legal Data and Systems within Operate, for PricewaterhouseCoopers (PwC) in London where she focuses on leading the operational delivery of managed legal programmes at PwC. Within her role she is also responsible for supporting clients and colleagues in navigating emerging technologies, such as GenAI.