Volker Matz and Martin Cox outline some of the obstacles facing organisations seeking to use generative AI in their processes.
Generative AI is at the forefront of current and future organisational change, offering significant opportunities for innovation but also posing challenges around adoption, copyright, and regulatory compliance. As businesses integrate AI-generated content into their operations, it is crucial to address legal considerations such as intellectual property rights, data privacy, and ethical AI use. A structured approach to AI implementation, focusing on foundation model selection, data protection, and compliance with regulations like the General Data Protection Regulation and emerging AI laws, is essential. Equally important is the role of Change Management in guiding organisations through the impacts on employees and operations, managing risks related to bias, accountability, and evolving work conditions. Effective collaboration between legal, technical, and leadership teams is needed to ensure a secure, compliant, and sustainable AI adoption, aligning with best practices and legal standards.
We are both active Transformational Change practitioners, so unsurprisingly, Generative AI is the topic at the forefront of current and upcoming organisational change. The present enthusiasm among clients has fostered a significantly more positive mindset towards technological innovation, including some technologies that have been available for several years.
While this recent surge in transformational change is welcome news for practitioners, technology firms, and change consultancies, the challenges of adopting and implementing Generative AI remain and are a frequently discussed topic for discussion.
With the increasing adoption of Generative AI in business practices, new content—such as images, music, and videos—can now be created within seconds. While this capability is impressive, it raises important questions about managing content ownership. Although AI-generated content is new, it often relies on training data from existing sources, which can lead to unintended copyright infringements, unclear attributions, or other legal uncertainties. A thorough understanding of these issues is crucial for both users and creators of AI-generated content, as it helps navigate associated risks and ensures compliance with copyright laws. Behavioural and process-driven considerations, along with strategies to mitigate legal risks, should therefore be implemented.
Behavioural Considerations
A cautious, informed, and ethical approach to using Generative AI is essential. By prioritising these adjustments, users can better navigate the complexities of copyright and intellectual property law while tapping into the creative potential of AI. Before using AI-generated content, users should understand the datasets used to train the AI model, including any licensing restrictions or references to copyrighted materials. This process may require a considerable time investment and can be complex. To streamline this, users might select AI models trained on datasets in the public domain or licensed under Creative Commons, ensuring the resulting content does not infringe copyright.
Responsible AI usage practices include attributing sources when AI-generated content is based on identifiable works, even if not legally required. For commercial purposes, seeking legal advice before deploying AI-generated content is advisable, especially when the origins of training data are unclear. Direct reproduction of existing works through Generative AI, even with modifications, should be avoided.
Process-Driven Considerations
Establishing internal procedures to ensure that AI-generated content complies with copyright laws is essential for maintaining compliance. These procedures help businesses adhere to intellectual property laws and minimise the risk of accidental copyright breaches. This approach not only reduces legal risks but also supports the responsible use of Generative AI within a framework that respects the rights of original content creators.
To ensure effective and compliant adoption of Generative AI, it is essential to address technical, ethical, and regulatory challenges through a structured, legally sound approach to implementation and scaling.
Foundation Model Selection and Architecture
Selecting an AI foundation model requires careful consideration of intellectual property rights, data security, and regulatory compliance, such as the General Data Protection Regulation. Protecting proprietary AI outputs is critical, and legal teams must ensure compliance with copyright laws like the EU Directive 2001/29/EC, which regulates the use of copyrighted content in AI training and outputs.
Vendor agreements should clarify intellectual property ownership, particularly regarding AI- generated content. Although legal debates around AI-generated works continue, organisations must establish clear terms on intellectual property rights in vendor contracts, ensuring alignment with international standards.
Model Access and Data Protection
Accessing Generative AI models, whether through public clouds or managed services, brings concerns about data privacy and security. This includes compliance with regulations like the General Data Protection Regulation and emerging frameworks such as the EU Artificial Intelligence Act. Secure data management and adherence to global privacy laws ensure that organisations’ data processing agreements align with Article 28 of the General Data Protection Regulation, addressing data handling, access rights, and retention periods.
Ethical frameworks guide the development of AI models in compliance with regulations like the UK Data Protection Act 2018, ensuring robust privacy and security measures.
Adapting Models with Proprietary Data
Integrating proprietary data into AI models involves significant risks related to confidentiality, data privacy, and ownership. This requires consideration of regulations like the General Data Protection Regulation’s Data Minimisation Principle (Article 5). Emphasising reinforcement learning helps organisations maintain data governance, ensuring that proprietary data used in AI training aligns with General Data Protection Regulation’s Article 32 (Security of Processing), including encryption and access controls. Legal departments must ensure that proprietary data usage complies with intellectual property laws and the Trade Secrets Act to prevent unauthorised disclosure.
Enterprise Readiness and AI Compliance
Ensuring enterprise readiness for Generative AI requires comprehensive compliance frameworks that address AI ethics, regulatory obligations, and industry-specific standards like the EU General Product Safety Directive. Focusing on compliance management enables legal teams to build governance structures, ensuring adherence to regulations such as the EU AI Act, which classifies AI systems by risk and sets specific legal requirements for “high-risk” AI systems.
Legal teams should leverage frameworks to ensure compliance with ethical AI standards, including the Ethics Guidelines for Trustworthy AI by the European Commission’s High-Level Expert Group on AI.
Industrialisation of Generative AI Applications
Scaling AI applications introduces risks of IP infringement, AI liability, and compliance with evolving AI-specific regulations like the EU AI Liability Directive and the UK’s National AI Strategy. Contracts should consider AI liability under emerging laws like the EU AI Liability Directive, which addresses compensation for damages caused by AI systems. Business frameworks assist legal teams in structuring contracts that protect IP rights and minimise risks of AI-generated outputs under laws for artistic and literary work protection.
Scaling AI Operations and Cross-Functional Legal Collaboration
Cross-functional collaboration involves legal challenges, such as data sharing, privacy, and compliance with regulations like the General Data Protection Regulation and Data Governance Act in the EU. Legal teams should establish secure data-sharing agreements, ensuring protection between departments and vendors. AI frameworks support risk management, ensuring collaboration aligns with regulatory standards. Legal teams should enable open innovation while adhering to sector-specific laws, like MiFID II for financial institutions, to ensure legally sound AI innovations.
Pilot Projects and Legal Safeguards
Pilot projects provide a way to assess legal and regulatory risks of Generative AI systems before full deployment, ensuring compliance with laws such as the EU AI Act. Hands-on learning from academic sources can equip legal professionals to assess risks related to algorithmic accountability frameworks. Documenting pilot project results ensures adherence to sector-specific regulations, such as the Financial Conduct Authority’s guidelines on AI in financial services. By incorporating these insights, legal teams can develop scalable frameworks that adapt to evolving AI technologies, ensuring ongoing compliance.
Broader Change Environment Beyond Technical Deployment
Transformational change extends beyond the technical deployment of solutions, emphasising the impact on people and organisations. This focus is critical for managing the employment and legal implications of Generative AI. Effective Change Management includes creating a clear vision for change, aligning with the organisation’s strategy, and guiding the transition through robust evaluation of progress. Specific attention should be given to how Generative AI affects bias, privacy, employee accountability, and evolving work conditions.
For organisations, it is crucial to seek expert legal guidance on areas such as contract law impacts, risk assessment, data privacy, and the management of employees’ personal data within AI models. A clear understanding of the implications for organisational health and safety, compliance with industry regulations, and ethical standards is essential for navigating the complexities of Generative AI deployments.
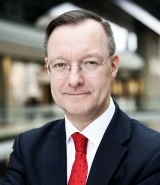
Volker Matz, Transformation Services, Data, Tech and AI
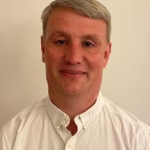
Martin Cox, Transformation Services, Data, Tech and AI